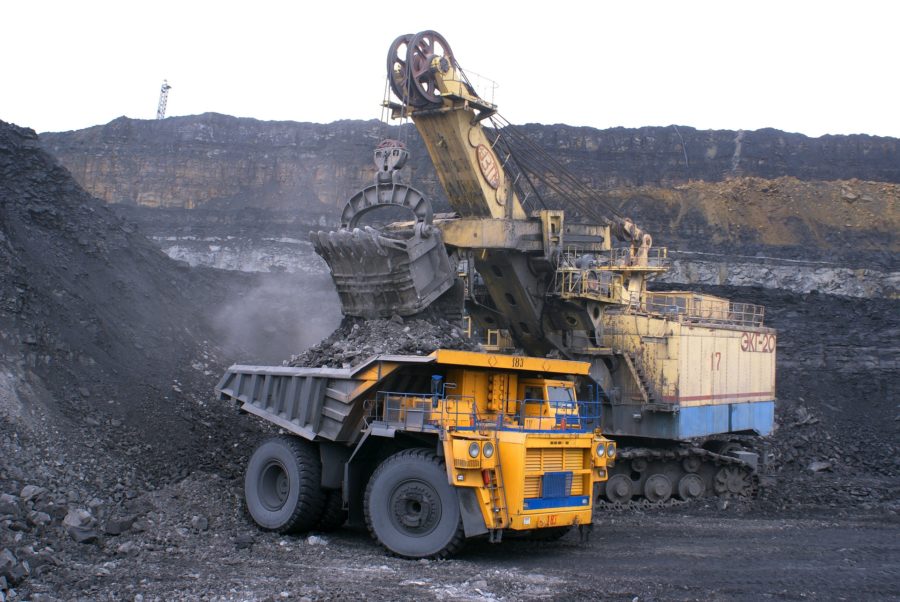
Emma White
Mention the word modelling and minds tend to drift towards washboard abs, perfect teeth and catwalks – the flow of groundwater – not so much. But modelling is a critical tool for managing supplies of underground water and assessing the potential impacts of large resource developments like coal mines. If we get it wrong, bad things happen – groundwater is contaminated, river flows decline, wetlands dry out, and ecosystems can lose the water they depend on.
The problem is that a model is only as good as the necessarily limited data it is based upon. “Garbage in, garbage out” as my Hawaiian-shirt wearing professor used to say sagely. Best available practice therefore relies on what we call “uncertainty analysis” which quantifies the likelihood that something may happen, but in Australia such analysis isn’t legally required. As a result, decision makers are not equipped with an accurate representation of risks and consequences. At best this leaves them blind to risk, and at worst, provides a false sense of security against more negative, but equally plausible outcomes.
For example, a 2015 decision by the Queensland Land Court to uphold approval for Adani to build the Carmichael Coal Mine despite concerns about impacts on groundwater, and particularly nearby Doongmabulla Springs, has been re-examined in a recent paper by researchers involved in the court challenge. The authors discuss modelling deficiencies and highlight the existence of an alternate and equally likely geological interpretation to that used by Adani. Adani proposed that the source of the springs is above a geological layer called the Rewan Formation while the alternate interpretation places the source below it. This is significant because if the source is below the Rewan Formation, Adani concedes the springs will be lost. And yet, Adani chose to use the best case scenario in their modelling and failed to explore the alternate interpretation.
So, how can this happen?
In hydrogeology, we measure things like the porosity (how much fluid a rock can hold) and hydraulic conductivity (how well fluid flows through the subsurface) at only a few spots in a study area, like in wells, drilling core samples, and at exposed rock outcrops. These spot locations are then used to extrapolate what is underground across the whole area – a bit like a dot-to-dot puzzle. But sometimes it can be akin to trying to sketch a person when all you can see is their baby toe. That is to say, we just don’t know for sure. As a result, geologists must use professional judgement to make an interpretation of the subsurface that’s consistent with the limited field data.
Available data is then used to build a conceptual geological model. The real world complexity cannot be completely captured by a model, so the most important structures such as layers, folds, faults are represented in a simplified way – a mannequin compared to a living breathing person. The conceptual geological model is then converted to a mathematical model using an equation that describes the flow of groundwater based on principles such as ‘water flows downhill’ and ‘things can’t be created out of nothing’.
What could possibly go wrong?
Well, firstly, if the conceptual model is incorrect then the predictions are meaningless. Hydrogeologists call this non-uniqueness. It basically means that while a conceptual model may fit the data, it may not be the only valid geological interpretation. There is no golden slipper interpretation for a Cinderella model. Consideration of non-uniqueness is vital for rigorous modelling.
Secondly, there is always a little twinge of doubt in all aspects of groundwater modelling (the underlying assumptions, the hydraulic properties of the soil and rock, and the predictions) that we call uncertainty. Too often, this level of uncertainty isn’t conveyed to people making the decisions, or worse, to people impacted by the decisions. It is meaningful to estimate the likelihood a predicted fall in water levels will occur based on current knowledge, instead of pinning-the-tail-on the best-looking number.
In modelling terms predicting a water level decline down to the exact centimetre, as frequently occurs, isn’t just shooting an apple on a kid’s head from a couple of yards away, but shooting it from the other side of the paddock, after spinning wizzy-dizzys for a good half hour. That level of precision is unheard of in groundwater modelling. The uncertainty surrounding a predicted value needs to be conveyed and understood.
Amassing data is the best way to reduce uncertainty and yet, there were significant data gaps in the groundwater model used for the Adani Carmichael Approval that were recognised and acknowledged by all hydrogeological experts involved in the Land Court Challenge. These data gaps included the source, flow rate and degree of impact upon the springs, faulting, geological interpretation, water chemistry, water levels and topographic data. This was uncertainty on a Ben Hur kind of scale.
But still, the testimonies of expert hydrogeologists were unable to convince the Land Court of the importance of uncertainty in the modelling process. Nor could a report from an independent expert panel, tasked with assessing large scale coal and coal seam gas projects, which highlighted the gaping gaps, considerable uncertainty, and questioned the legitimacy of the model conceptualisation.
This is a fundamental failing in our approval process as you can’t make a defensible model without data. Data collection and uncertainty resolutions should occur before project approval so that all data required to make an informed decision on the potential impact of the development can be considered. The onus must be on the proponent to prove beyond a reasonable doubt that the development will not have adverse impacts on groundwater levels and dependent ecosystems.
Our scientific approval process should be based on science, not emotion or rhetoric. Modelling is valuable, but a rigorous approvals process is more valuable. It would benefit everyone to remember that, ‘all models are wrong, but some are useful’.
Because when a model is good, it is very, very, good, but when it is bad, it is horrid.